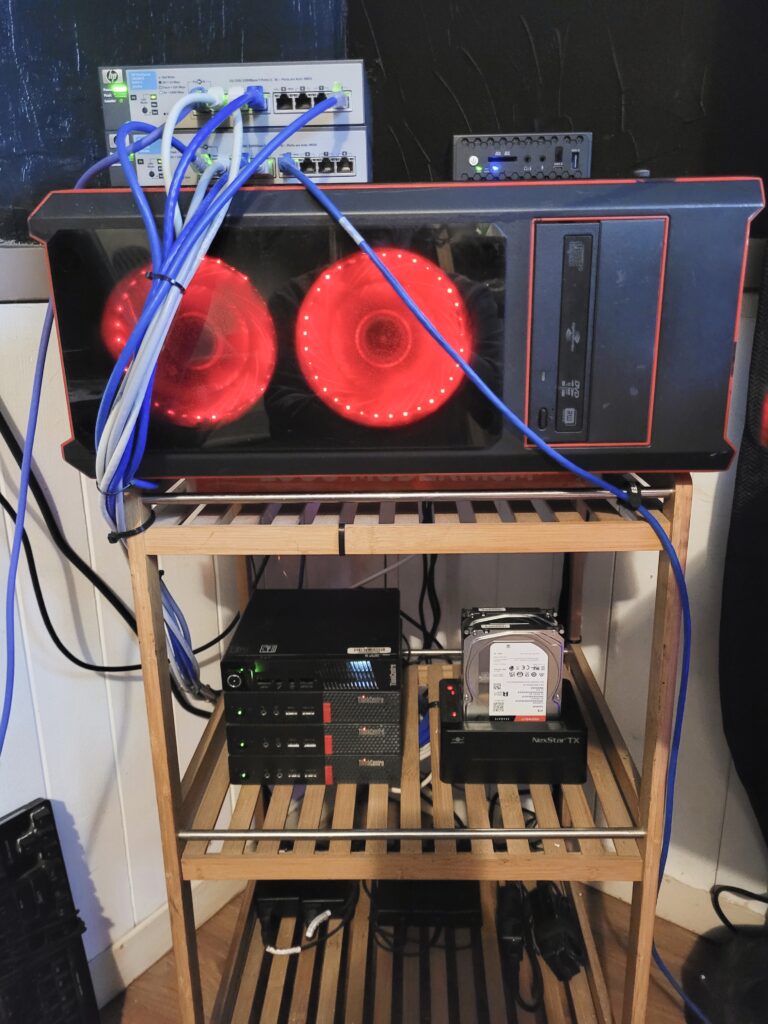
As my homelab continues to evolve, I’m excited to share the latest addition: a GPU-capable Proxmox node purpose-built for local AI workloads. In this post, I’ll walk you through the motivation behind the upgrade, the hardware and software setup, and how it ties into my existing infrastructure—featuring my custom home lab portal, a robust Prometheus/Grafana monitoring stack, and VisionMapr, my mind-mapping web app.
🎥 Watch the Setup in Action
Below is a short video walk through where I discuss the new node, demonstrate GPU pass-through in Proxmox, and give you a peek at the portal, monitoring dashboards, and VisionMapr in action. Feel free to skip ahead to any section that interests you!
Hardware Overview
All of my ThinkCentre mini PCs in the cluster are Lenovo M710Q models, handling general workload and virtualization duties. The brand-new Proxmox node brings dedicated GPU horsepower to the mix:
- CPU: AMD FX-6300 (6 cores, 6 threads)
- RAM: 32 GB DDR3
- GPU: NVIDIA GTX 1060 (6 GB VRAM)
This configuration strikes a balance between cost, power consumption, and performance for experimenting with on-premise AI models.
Why GPU Passthrough?
Traditional KVM virtualization handles CPU and RAM with ease, but GPU acceleration is essential for:
- Model Inference: Running neural networks at usable speeds
- Parallel Workloads: Leveraging CUDA cores for batch processing
- Future Proofing: Supporting frameworks like TensorFlow, PyTorch, and LLaMA-style models
Enabling GPU passthrough ensures my AI containers can access the GTX 1060 directly, avoiding the overhead of software emulation.
Local AI with Open WebUI & Olama
The crown jewel of this upgrade is the ability to run LLMs entirely on-premise:
- Open WebUI
- A browser interface for loading models, setting prompts, and visualizing outputs.
- Olama
- A fast, Rust-based inference engine that leverages CUDA cores on the GTX 1060.
- Use Cases
- Code generation for my Unity and PHP projects.
- Automated blog post summaries.
- Experimentation with fine-tuning small models against my personal data.
This setup gives me full data privacy, zero recurring cloud costs, and instant iteration loops.
Next Steps
Adding GPU capability to my Proxmox cluster marks a major milestone in my homelab journey. With the AMD FX-6300 and GTX 1060 now online, I’m empowered to:
- Train and serve AI models locally
- Enhance monitoring with GPU-aware dashboards
- Integrate accelerated web apps like VisionMapr
Stay tuned for deeper dives into fine-tuning workflows, advanced Proxmox networking, and performance benchmarks. In the meantime, let me know in the comments what AI experiments you’d like to see next!